Machine Learning
As astronomers build increasingly larger observatories capable of seeing more objects in the sky, the amount of data they collect has gone beyond what humans can analyze without help. Instead, researchers turn to teaching computers to sift through the data, identifying important patterns and connections that might otherwise be missed. This process is called machine learning, and it’s an essential aspect of modern astronomy at the Center for Astrophysics.
Our Work
Center for Astrophysics | Harvard & Smithsonian scientists and engineers use machine learning in many different ways:
-
Identifying and classifying objects and transient events within large surveys of the sky. Those surveys include current projects such as the Baryon Oscillation Spectroscopic Survey (BOSS) and upcoming observatories like the Large Synoptic Survey Telescope (LSST). LSST in particular will produce as much as 20 terabytes per night.
A One-Percent Measure of Galaxies Half the Universe Away -
Using machine learning to study day-to-day changes in the Sun, in an effort to understand solar weather. The space-based Solar Dynamics Observatory (SDO) collects 1.5 terabytes of data daily to ensure as detailed a picture as possible. Computer algorithms help sort this data and identify important features, such as solar flares.
Solar Dynamics Observatory -
Identifying exoplanets using data from the Kepler/K2 observatory. This space telescope has collected years of data on more than 150,000 stars to find the tiny flickers indicating the presence of planets. Machine learning helps separate signs of planets from other fluctuations in light form those stars, as well as identifying exoplanets that would be hard to spot otherwise.
Artificial Intelligence, NASA Data Used to Discover Eighth Planet Circling Distant Star -
Hunting for transient events like supernovas and the light-producing counterparts to gravitational wave discoveries. These events are some of the most powerful cosmic cataclysms, but they last only for a relatively short time before fading. That means identifying and studying them as quickly as possible. Machine learning helps by automating the process of finding these transient events within other data, including observations where finding supernovas wasn’t the original intent.
Astronomers See Light Show Associated With Gravitational Waves
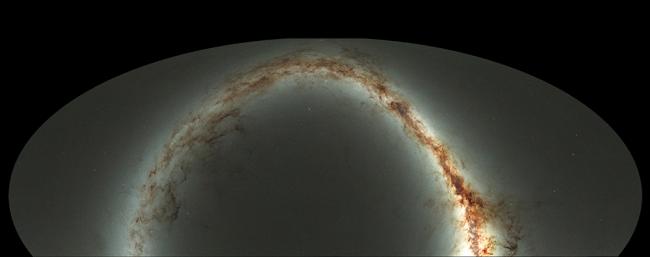
This view of the sky seen by the Pan-STARRS1 telescope in Hawaii contains 3 billion astronomical sources, ranging from nearby asteroids to distant galaxies. Researchers use machine learning techniques to help analyze the 2 petabytes of data collected.
A Universe of Data
Machine learning plays a huge role when cataloguing large numbers of anything, like galaxies in surveys of the whole sky. Computers can learn to identify and classify galaxy types, find transient events like supernovas, and pick out features in galaxy clusters. With thousands of potential sources from large surveys, it would take humans far too much time which could be better spent on other tasks. Machine learning shifts the effort from astronomers to computers, which excel at tedious detail-oriented tasks. Computer algorithms can also apply what we’ve learned from high-resolution observational data to improve lower-resolution images, allowing astronomers to construct what that object might look like through a more powerful telescope.
In addition, machine learning is essential for “time-domain astronomy”: looking for events that change during observation. Those include:
-
Hunts for exoplanets, which are planets orbiting other stars. When these worlds pass between their host stars and Earth, they block a small amount of light. Tracking the duration and amount of light provides information about the planet’s size and orbit. Several exoplanets have been identified using machine learning, including a few in multiple-planet systems, where the signals are hard for a human to distinguish.
-
Tracking changes in the light from stars. Some stars are extremely “active”, producing flares at unpredictable intervals. Others are variable, changing brightness as they expand and contract. Computers are suited to catch these variations, which can be subtle compared with the sheer amount of data needed to find them.
-
Studying patterns in the “weather” on our own Sun. Multiple observatories monitor the Sun literally all the time, which produces a vast amount of data to sift through. Machines are very good at finding important fluctuations in the Sun’s activity within that mass of information.
-
Finding and classifying supernovas. The explosions of stars and white dwarfs are random, unpredictable occurrences for all practical purposes. Catching these transient events requires sifting through data that’s often collected for different purposes, such as catalogs of galaxies.
-
Locating asteroids, comets, and other faint objects in the Solar System. These bodies show up as transients in other astronomical images. Computer searches are well suited to find them in large catalogs of data.
-
Using machine learning to classify objects in astronomical images. For example, in a telescope image of a galaxy or cluster of stars, the resolution might not be good enough to distinguish individual stars. However, computer processing can pick out light from different stars in the population, similar to the way low-resolution photos can be sharpened to reveal details that can’t be seen in the original.
Both current and future observatories regularly process many terabytes — trillions of bytes — of data. With that much information, it can be hard to tell what is important and what is not, with the importance depending on what scientific questions are being asked. Astronomers can teach computers to process the flood of data to pick out those important pieces.
Many current and future observatories, including NASA’s Transiting Exoplanet Survey Satellite (TESS), will bring in even more data useful to many areas of research. As a result, machine learning will become more important in the coming years, and CfA scientists are on the cutting edge of that development.
Related News
Giant Magellan Telescope Mount Fabrication Begins
The Giant Magellan Telescope’s Final Mirror Fabrication Begins
Billions of Celestial Objects Revealed in Gargantuan Survey of the Milky Way
The Most Precise Accounting Yet of Dark Energy and Dark Matter
Dozens of Newly Discovered Gravitational Lenses Could Reveal Ancient Galaxies and the Nature of Dark Matter
Artificial Intelligence Classifies Supernova Explosions with Unprecedented Accuracy
Projects
AstroAI
Seamless Astronomy
Telescopes and Instruments
Giant Magellan Telescope
Visit the GMT Website
Lynx X-Ray Observatory
Visit the Lynx X-Ray Observatory Website
Magellan Telescopes
Visit the Magellan Telescopes Website
MMT Observatory
Visit the MMT Website
Spitzer Space Telescope
Visit the Spitzer Space Telescope IRAC Page